Table of Contents
SECTION 1
Question 1
a)
Table 1. Descriptive Statistics: Measure of Dispersion and Central Tendency
Measures | Statistics |
N | 12 |
Mean | 2.125 |
Median | 2.05 |
Mode | -5.40a |
Std. Deviation | 4.912 |
Variance | 24.13 |
Range | 15.8 |
Minimum | -5.4 |
Maximum | 10.4 |
- b)
Based on the central tendency results, it is observed that the mean of industry’s sales growth is greater than the median and this implies that the data could be right skewed. The mode is the data is seen to be -5.4 and this means that the distribution’s overall shape is unimodal.
In terms of dispersion, it is pertinent to note that the smaller the number or value of dispersion, the more the consistence of the data and thus, the better the predictions. The range calculated is seen to be 15.8 and this value is suggested to be relatively big and thus, the industry’s sales growth largely spread out. The standard deviation(4.912) obtained is seen to be relatively high and the spread of the growth rates of sales of the companies in the year 2015 from the mean growth rates of sales is seen to be relatively poor. The variance of the growth rates of sales for 2015 (24.13) is considered to be relatively high and this implies that there is a very high spread out of the data points from the given growth rate mean as well as from each other. This implies that the growth rate of sales data for 2015 is not consistent and the predictions to be made will not be accurate.
Question 2
a)
The statistical method to be used in assessing the observation is Probability of normal distribution
b)
The probability that the shares of the company are greater than 300 is calculated as below:
The population mean: 221
The standard deviation: 1.6
PX>300=P(Z>300-2211.6)
=P(Z>49.375
=0.5+0.49
=0.99
b)
The probability that the company is less than 55 is calculated as below:
PX>300=P(Z<55-2211.6)
=P(Z>-103.75)
=P(Z>103.75)
=0.5+0.499
=0.99
Question 3:
a)
The null and alternative hypothesis was formulated as below:
Null Hypothesis:
There is no statistically significant relationship between employee’s productivity and the investment amount on employee’s training
Alternative Hypothesis:
There is statistically significant relationship between employee’s productivity and the investment amount on employee’s training
b)
The Pearson’s Correlation Coefficient between productivity and investment on training was calculated as below:
Table 2. Pearson’s Correlation between Investment in Training and Output per Head
Output per Head | Investment in Training per Head | ||
Output per Head | Pearson Correlation | 1 | .425 |
Investment in Training per Head | Pearson Correlation | .425 | 1 |
Based upon Table 2, it is evident that the Pearson’s Correlation Coefficient between output per head and investment in training is 0.425.
c)
The correlation coefficient statistical significance was determined using t-test as below:
Table 3. Correlation Coefficient Statistical Significance
Output per Head | Investment in Training per Head | ||
Output per Head | Pearson Correlation | 1 | .425 |
Sig. (2-tailed) | .169 | ||
Investment in Training per Head | Pearson Correlation | .425 | 1 |
Sig. (2-tailed) | .169 |
The p value is observed to be 0.169 and it is greater than 0.05 thus, this means that the correlation coefficient is not statistically significant.
d)
The distinction between causation and correlation can be described as below:
Based upon the results in Table 2 and 3, it is evident that the correlation coefficient is 0.425 and the p value is 0.169.The p value is seen to be greater than 0.05 and this implies that the null hypothesis is not rejected at 0.05 level of significance and hence, there is no statistically significant relationship between employee’s productivity and the investment amount on employee’s training. It can therefore, be concluded that investments made in training have no significant impact on the productivity of employees. This is further supported by the relatively low correlation of coefficient which indicates a weak relationship between productivity of employees and investment in training.
e)
The advice to be given to companies that would wish to increase the workforce productivity is that:
Investing in training of employees is not the best option of increasing workforce productivity and this is based on the fact that there is no enough evidence to prove that taskforce productivity is influenced by training of the employees. Thus, companies should consider other factors which could have a significant effect on taskforce productivity.
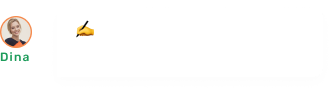
SECTION 2
Question 4
a)
Regression method was used in the estimation of β1 and β2 as below:
The below linear equation was used and it assumes the relationship between Disposable Income(Y) and Consumer Expenditure(C).
Ct = β1 + β2Yt + ut
Table 4. The Estimation of β1 and β2
Coefficients | Sig (at 5%) | Std. Error | ||
1 | Constant | 598.480 | .011 | 193.276 |
Disposable Income | .763 | .000 | 0.016 |
Based upon Table 4, it is evident that β1 and β2 are 598.48 and 0.763 respectively.
b)
In terms of the constant coefficient estimate, it can be concluded that constant coefficient is statistically significant and this is because its p value (0.011) is less than 0.05. The constant is as well seen to be extremely high.
c)
In terms of the slope coefficient estimate β2 , it is observed that it is positive and relatively high as well as statistically significant since its p value (0.00) is less than 0.05. These results mean that disposable income has a positive influence on consumer expenditure. Thus, a unit changes in disposable income causes 76.3% change in consumer expenditure.
d)
The R-squared of the model is observed to be 0.995 and this value means that:
The 99.5% variation of consumer expenditure is explained by the disposable income. This as well means that the goodness of fit is excellent.
e)
The results of the model are seen to be accurate and statistically significant. However, this model can be improved by considering other confounding factors which do have an influence on consumer expenditure. The model can be improved by transforming the data points and thus, natural logs could be used and by so doing this, variation and consistence in the data set is reduced and improved respectively.
SECTION 3
a)
The Labor Statistics Bureau noted that about 77% of women earned more salary than men in the year 1999(Webbink & Hartog 2004). Despite the fact that there is proper documentation of gender pay gap, the factors leading to gender pay gap are still not clear. Variation on the level of jobs associated with men and women is one of these factors. However, exploring how job variation influences the gap between the earning of men and women can be further complex when both women and men in female dominated occupations are paid less than women and men in a male prevalent occupation.
The reason why occupations associated with females seems to pay less has never been clear. There are different factors which have been suggested to affect this and they are such as discrimination, women society devaluation, occupational crowding, job type and the characteristics of workers. The current analysis explores different aspects related to the existence of gender gap earnings. The literature notes that there was a negative relationship between women share in a given occupation and wages and as such, the characteristics of job and worker were controlled. The literature has identified various factors that influence future earnings expectations and they include information sources, age, gender and socioeconomic background (Webbink & Hartog 2004). Previous studies have identified the earning gap between females and males (Chevalier 2004). Thus, it is believed that gender earnings expectations are affected by this observation. The study carried out by Chevalier noted that women earns between 20% and 40% less than men regardless of the existence of a law on equal opportunity. It was explained that the earning gap between male and female was as a result of gender variations in career choices, education and expectations. Different studies have as well highlighted that men expect to earn more than women (Mazza & Hartog 2011). Age influence on earnings has been exploited by several studies (Brunello et al. 2004). The study reported that the expectation of earnings between senior and junior students was similar. Other similar studies found different results where the expectations of earnings for senior students were higher when compared to that of junior students.
b)
The literature notes that gender has an influence on earnings where it is believed men earn more than women based on different factors such as discrimination
Null Hypothesis:
There is no statistically significant relationship between gender and earnings
Alternative Hypothesis:
There is statistically significant relationship between gender and earnings
The literature also identifies various factors that influence future earnings expectations and they include information sources, age, education, gender and socioeconomic background, but for this study we consider age and education. In this case, the study attempts to investigate how age and education affects earnings.
Null Hypothesis:
There is no statistically significant relationship between Education and earning gap between genders
Alternative Hypothesis:
There is statistically significant relationship between Education or Age and earnings gap between genders
The equation model used in testing the above hypotheses is illustrated below and the dependent variable considered was earnings. The independent variables considered include age, education and gender.
EARNINGS = b1 + b2MALE +b3AGE + b4EDUCBA
Figure 5 represents multiple linear regression analysis of the relationship between age, gender, education and earnings. The aim of the analysis was to investigate if age and education have an effect on the gap earnings and thus testing the hypothesis whether there is statistically significant relationship between Education, age and gender and earning gap in terms of gender.
Table 5. Linear Regression for Age, Education, gender and Earnings Relationship
Model | Coefficients | t | Sig. | ||
B | Std. Error | ||||
1 | (Constant) | 12.777 | 10.262 | 1.245 | .214 |
MALE | 6.776 | 1.158 | 5.850 | .000 | |
EDUCBA | 10.062 | 1.464 | 6.872 | .000 | |
AGE | .037 | .250 | .148 | .882 |
Based upon Figure 5, it is observed that male and education have coefficients (6.78 and 10.062 respectively) that are statistically significant. In this case, it is observed that their p values are less than 0.05. Furthermore, both of their coefficients are positive. Based upon this, the null hypothesis that there is no statistically linear relationship between gender and earnings as well as education and earnings is rejected and the conclusion made is that education and gender have a positive linear relationship with earnings. Specifically, a unit increase in education level leads to 10 units increase in earnings and also, a unit increase in gender difference will lead to 6.7 unit increase in earnings. On the other hand, the constant and age have coefficients that are not statistically significant and this means that the null hypothesis which states that there is no statistically significant linear relationship between age and earnings is not rejected. The conclusion made here is that there is no enough evidence to prove that age have a linear relationship with earnings of age has influence on earnings. The R-squared is seen to be 13.5% and this means that 13.7% of earnings variations are caused by age, gender and education and thus the goodness of good fit is considered to be relatively poor.
d)
Diagnostic test was undertaken as seen in Table 6 and in this case it entailed multicollinearity,
Table 6. Collinearity Statistics for Age, Education, Gender and Earnings
Model | Collinearity Statistics | ||
Tolerance | VIF | ||
1 | MALE | .998 | 1.002 |
EDUCBA | .997 | 1.003 | |
AGE | .996 | 1.004 |
It can be stated that VIF which is greater than 3 indicates that there is collinearity between a given variables. Based upon Table 6, it is observed that the collinearity values for male, education and age are 1.002, 1.003 and 1.004 respectively. This means that the independent variables which are age, education and gender have no collinearity issues with the dependent variable which is earning. The limitation is that the more you have many independent variables the more you experience multicollinearity issues. Another limitation of the study is that not all independent variables were considered in the analysis and yet they have been identified by the literature to have a significant effect on earnings and they are such as socioeconomic characteristics. Another limitation of the results is that data transformation was not carried out and yet it is very important in removing outliers which are normally associated with results bias and hence, leading to inaccurate results. These results do not clearly justify previous studies which identified the earning gap between females and males (Chevalier 2004). Thus, it is believed that gender earnings expectations are affected by this observation. This is because the earning data was not specific to earning gaps between genders.
- Webbink, Dinand and Joop Hartog, 2004, “Can Students Predict their Starting Salary? Yes!”, Economics of Education Review, 23(2), pp. 103-113.
- Mazza, Jacopo and Joop Hartog, 2011, “Do They Understand the Benefits From Education? Evidence on Dutch High School Students’ Earnings Expectations”, IZA Discussion Paper, No. 5714, Bonn: Institute for the Study of Labor.
- Chevalier, Arnaud, 2004, “Motivation, Expectations and the Gender Pay Gap for UK Graduates”, IZA Discussion Paper, No. 1101, Bonn: Institute for the Study of Labor.
- Brunello, Giorgio, Claudio Lucifora and Rudolf Winter-Ebmer, 2004, “The Wage Expectations of European Business and Economics Student”, Journal of Human Resources, 39(4), pp. 1116-1142.